mirror of
https://github.com/davisking/dlib.git
synced 2024-11-01 10:14:53 +08:00
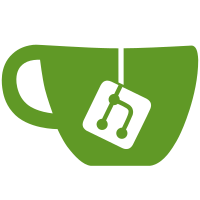
* Add concat_prev layer, and U-net example for semantic segmentation * Allow to supply mini-batch size as command-line parameter * Decrease default mini-batch size from 30 to 24 * Resize t1, if needed * Use DenseNet-style blocks instead of residual learning * Increase default mini-batch size to 50 * Increase default mini-batch size from 50 to 60 * Resize even during the backward step, if needed * Use resize_bilinear_gradient for the backward step * Fix function call ambiguity problem * Clear destination before adding gradient * Works OK-ish * Add more U-tags * Tweak default mini-batch size * Define a simpler network when using Microsoft Visual C++ compiler; clean up the DenseNet stuff (leaving it for a later PR) * Decrease default mini-batch size from 24 to 23 * Define separate dnn filename for MSVC++ and not * Add documentation for the resize_to_prev layer; move the implementation so that it comes after mult_prev * Fix previous typo * Minor formatting changes * Reverse the ordering of levels * Increase the learning-rate stopping criterion back to 1e-4 (was 1e-8) * Use more U-tags even on Windows * Minor formatting * Latest MSVC 2017 builds fast, so there's no need to limit the depth any longer * Tweak default mini-batch size again * Even though latest MSVC can now build the extra layers, it does not mean we should add them! * Fix naming
244 lines
12 KiB
C++
244 lines
12 KiB
C++
// The contents of this file are in the public domain. See LICENSE_FOR_EXAMPLE_PROGRAMS.txt
|
|
/*
|
|
Semantic segmentation using the PASCAL VOC2012 dataset.
|
|
|
|
In segmentation, the task is to assign each pixel of an input image
|
|
a label - for example, 'dog'. Then, the idea is that neighboring
|
|
pixels having the same label can be connected together to form a
|
|
larger region, representing a complete (or partially occluded) dog.
|
|
So technically, segmentation can be viewed as classification of
|
|
individual pixels (using the relevant context in the input images),
|
|
however the goal usually is to identify meaningful regions that
|
|
represent complete entities of interest (such as dogs).
|
|
|
|
Instructions how to run the example:
|
|
1. Download the PASCAL VOC2012 data, and untar it somewhere.
|
|
http://host.robots.ox.ac.uk/pascal/VOC/voc2012/VOCtrainval_11-May-2012.tar
|
|
2. Build the dnn_semantic_segmentation_train_ex example program.
|
|
3. Run:
|
|
./dnn_semantic_segmentation_train_ex /path/to/VOC2012
|
|
4. Wait while the network is being trained.
|
|
5. Build the dnn_semantic_segmentation_ex example program.
|
|
6. Run:
|
|
./dnn_semantic_segmentation_ex /path/to/VOC2012-or-other-images
|
|
|
|
An alternative to steps 2-4 above is to download a pre-trained network
|
|
from here: http://dlib.net/files/semantic_segmentation_voc2012net_v2.dnn
|
|
|
|
It would be a good idea to become familiar with dlib's DNN tooling before reading this
|
|
example. So you should read dnn_introduction_ex.cpp and dnn_introduction2_ex.cpp
|
|
before reading this example program.
|
|
*/
|
|
|
|
#ifndef DLIB_DNn_SEMANTIC_SEGMENTATION_EX_H_
|
|
#define DLIB_DNn_SEMANTIC_SEGMENTATION_EX_H_
|
|
|
|
#include <dlib/dnn.h>
|
|
|
|
// ----------------------------------------------------------------------------------------
|
|
|
|
inline bool operator == (const dlib::rgb_pixel& a, const dlib::rgb_pixel& b)
|
|
{
|
|
return a.red == b.red && a.green == b.green && a.blue == b.blue;
|
|
}
|
|
|
|
// ----------------------------------------------------------------------------------------
|
|
|
|
// The PASCAL VOC2012 dataset contains 20 ground-truth classes + background. Each class
|
|
// is represented using an RGB color value. We associate each class also to an index in the
|
|
// range [0, 20], used internally by the network.
|
|
|
|
struct Voc2012class {
|
|
Voc2012class(uint16_t index, const dlib::rgb_pixel& rgb_label, const std::string& classlabel)
|
|
: index(index), rgb_label(rgb_label), classlabel(classlabel)
|
|
{}
|
|
|
|
// The index of the class. In the PASCAL VOC 2012 dataset, indexes from 0 to 20 are valid.
|
|
const uint16_t index = 0;
|
|
|
|
// The corresponding RGB representation of the class.
|
|
const dlib::rgb_pixel rgb_label;
|
|
|
|
// The label of the class in plain text.
|
|
const std::string classlabel;
|
|
};
|
|
|
|
namespace {
|
|
constexpr int class_count = 21; // background + 20 classes
|
|
|
|
const std::vector<Voc2012class> classes = {
|
|
Voc2012class(0, dlib::rgb_pixel(0, 0, 0), ""), // background
|
|
|
|
// The cream-colored `void' label is used in border regions and to mask difficult objects
|
|
// (see http://host.robots.ox.ac.uk/pascal/VOC/voc2012/htmldoc/devkit_doc.html)
|
|
Voc2012class(dlib::loss_multiclass_log_per_pixel_::label_to_ignore,
|
|
dlib::rgb_pixel(224, 224, 192), "border"),
|
|
|
|
Voc2012class(1, dlib::rgb_pixel(128, 0, 0), "aeroplane"),
|
|
Voc2012class(2, dlib::rgb_pixel( 0, 128, 0), "bicycle"),
|
|
Voc2012class(3, dlib::rgb_pixel(128, 128, 0), "bird"),
|
|
Voc2012class(4, dlib::rgb_pixel( 0, 0, 128), "boat"),
|
|
Voc2012class(5, dlib::rgb_pixel(128, 0, 128), "bottle"),
|
|
Voc2012class(6, dlib::rgb_pixel( 0, 128, 128), "bus"),
|
|
Voc2012class(7, dlib::rgb_pixel(128, 128, 128), "car"),
|
|
Voc2012class(8, dlib::rgb_pixel( 64, 0, 0), "cat"),
|
|
Voc2012class(9, dlib::rgb_pixel(192, 0, 0), "chair"),
|
|
Voc2012class(10, dlib::rgb_pixel( 64, 128, 0), "cow"),
|
|
Voc2012class(11, dlib::rgb_pixel(192, 128, 0), "diningtable"),
|
|
Voc2012class(12, dlib::rgb_pixel( 64, 0, 128), "dog"),
|
|
Voc2012class(13, dlib::rgb_pixel(192, 0, 128), "horse"),
|
|
Voc2012class(14, dlib::rgb_pixel( 64, 128, 128), "motorbike"),
|
|
Voc2012class(15, dlib::rgb_pixel(192, 128, 128), "person"),
|
|
Voc2012class(16, dlib::rgb_pixel( 0, 64, 0), "pottedplant"),
|
|
Voc2012class(17, dlib::rgb_pixel(128, 64, 0), "sheep"),
|
|
Voc2012class(18, dlib::rgb_pixel( 0, 192, 0), "sofa"),
|
|
Voc2012class(19, dlib::rgb_pixel(128, 192, 0), "train"),
|
|
Voc2012class(20, dlib::rgb_pixel( 0, 64, 128), "tvmonitor"),
|
|
};
|
|
}
|
|
|
|
template <typename Predicate>
|
|
const Voc2012class& find_voc2012_class(Predicate predicate)
|
|
{
|
|
const auto i = std::find_if(classes.begin(), classes.end(), predicate);
|
|
|
|
if (i != classes.end())
|
|
{
|
|
return *i;
|
|
}
|
|
else
|
|
{
|
|
throw std::runtime_error("Unable to find a matching VOC2012 class");
|
|
}
|
|
}
|
|
|
|
// ----------------------------------------------------------------------------------------
|
|
|
|
// Introduce the building blocks used to define the segmentation network.
|
|
// The network first does residual downsampling (similar to the dnn_imagenet_(train_)ex
|
|
// example program), and then residual upsampling. In addition, U-Net style skip
|
|
// connections are used, so that not every simple detail needs to reprented on the low
|
|
// levels. (See Ronneberger et al. (2015), U-Net: Convolutional Networks for Biomedical
|
|
// Image Segmentation, https://arxiv.org/pdf/1505.04597.pdf)
|
|
|
|
template <int N, template <typename> class BN, int stride, typename SUBNET>
|
|
using block = BN<dlib::con<N,3,3,1,1,dlib::relu<BN<dlib::con<N,3,3,stride,stride,SUBNET>>>>>;
|
|
|
|
template <int N, template <typename> class BN, int stride, typename SUBNET>
|
|
using blockt = BN<dlib::cont<N,3,3,1,1,dlib::relu<BN<dlib::cont<N,3,3,stride,stride,SUBNET>>>>>;
|
|
|
|
template <template <int,template<typename>class,int,typename> class block, int N, template<typename>class BN, typename SUBNET>
|
|
using residual = dlib::add_prev1<block<N,BN,1,dlib::tag1<SUBNET>>>;
|
|
|
|
template <template <int,template<typename>class,int,typename> class block, int N, template<typename>class BN, typename SUBNET>
|
|
using residual_down = dlib::add_prev2<dlib::avg_pool<2,2,2,2,dlib::skip1<dlib::tag2<block<N,BN,2,dlib::tag1<SUBNET>>>>>>;
|
|
|
|
template <template <int,template<typename>class,int,typename> class block, int N, template<typename>class BN, typename SUBNET>
|
|
using residual_up = dlib::add_prev2<dlib::cont<N,2,2,2,2,dlib::skip1<dlib::tag2<blockt<N,BN,2,dlib::tag1<SUBNET>>>>>>;
|
|
|
|
template <int N, typename SUBNET> using res = dlib::relu<residual<block,N,dlib::bn_con,SUBNET>>;
|
|
template <int N, typename SUBNET> using ares = dlib::relu<residual<block,N,dlib::affine,SUBNET>>;
|
|
template <int N, typename SUBNET> using res_down = dlib::relu<residual_down<block,N,dlib::bn_con,SUBNET>>;
|
|
template <int N, typename SUBNET> using ares_down = dlib::relu<residual_down<block,N,dlib::affine,SUBNET>>;
|
|
template <int N, typename SUBNET> using res_up = dlib::relu<residual_up<block,N,dlib::bn_con,SUBNET>>;
|
|
template <int N, typename SUBNET> using ares_up = dlib::relu<residual_up<block,N,dlib::affine,SUBNET>>;
|
|
|
|
// ----------------------------------------------------------------------------------------
|
|
|
|
template <typename SUBNET> using res64 = res<64,SUBNET>;
|
|
template <typename SUBNET> using res128 = res<128,SUBNET>;
|
|
template <typename SUBNET> using res256 = res<256,SUBNET>;
|
|
template <typename SUBNET> using res512 = res<512,SUBNET>;
|
|
template <typename SUBNET> using ares64 = ares<64,SUBNET>;
|
|
template <typename SUBNET> using ares128 = ares<128,SUBNET>;
|
|
template <typename SUBNET> using ares256 = ares<256,SUBNET>;
|
|
template <typename SUBNET> using ares512 = ares<512,SUBNET>;
|
|
|
|
template <typename SUBNET> using level1 = dlib::repeat<2,res64,res<64,SUBNET>>;
|
|
template <typename SUBNET> using level2 = dlib::repeat<2,res128,res_down<128,SUBNET>>;
|
|
template <typename SUBNET> using level3 = dlib::repeat<2,res256,res_down<256,SUBNET>>;
|
|
template <typename SUBNET> using level4 = dlib::repeat<2,res512,res_down<512,SUBNET>>;
|
|
|
|
template <typename SUBNET> using alevel1 = dlib::repeat<2,ares64,ares<64,SUBNET>>;
|
|
template <typename SUBNET> using alevel2 = dlib::repeat<2,ares128,ares_down<128,SUBNET>>;
|
|
template <typename SUBNET> using alevel3 = dlib::repeat<2,ares256,ares_down<256,SUBNET>>;
|
|
template <typename SUBNET> using alevel4 = dlib::repeat<2,ares512,ares_down<512,SUBNET>>;
|
|
|
|
template <typename SUBNET> using level1t = dlib::repeat<2,res64,res_up<64,SUBNET>>;
|
|
template <typename SUBNET> using level2t = dlib::repeat<2,res128,res_up<128,SUBNET>>;
|
|
template <typename SUBNET> using level3t = dlib::repeat<2,res256,res_up<256,SUBNET>>;
|
|
template <typename SUBNET> using level4t = dlib::repeat<2,res512,res_up<512,SUBNET>>;
|
|
|
|
template <typename SUBNET> using alevel1t = dlib::repeat<2,ares64,ares_up<64,SUBNET>>;
|
|
template <typename SUBNET> using alevel2t = dlib::repeat<2,ares128,ares_up<128,SUBNET>>;
|
|
template <typename SUBNET> using alevel3t = dlib::repeat<2,ares256,ares_up<256,SUBNET>>;
|
|
template <typename SUBNET> using alevel4t = dlib::repeat<2,ares512,ares_up<512,SUBNET>>;
|
|
|
|
// ----------------------------------------------------------------------------------------
|
|
|
|
template <
|
|
template<typename> class TAGGED,
|
|
template<typename> class PREV_RESIZED,
|
|
typename SUBNET
|
|
>
|
|
using resize_and_concat = dlib::add_layer<
|
|
dlib::concat_<TAGGED,PREV_RESIZED>,
|
|
PREV_RESIZED<dlib::resize_prev_to_tagged<TAGGED,SUBNET>>>;
|
|
|
|
template <typename SUBNET> using utag1 = dlib::add_tag_layer<2100+1,SUBNET>;
|
|
template <typename SUBNET> using utag2 = dlib::add_tag_layer<2100+2,SUBNET>;
|
|
template <typename SUBNET> using utag3 = dlib::add_tag_layer<2100+3,SUBNET>;
|
|
template <typename SUBNET> using utag4 = dlib::add_tag_layer<2100+4,SUBNET>;
|
|
|
|
template <typename SUBNET> using utag1_ = dlib::add_tag_layer<2110+1,SUBNET>;
|
|
template <typename SUBNET> using utag2_ = dlib::add_tag_layer<2110+2,SUBNET>;
|
|
template <typename SUBNET> using utag3_ = dlib::add_tag_layer<2110+3,SUBNET>;
|
|
template <typename SUBNET> using utag4_ = dlib::add_tag_layer<2110+4,SUBNET>;
|
|
|
|
template <typename SUBNET> using concat_utag1 = resize_and_concat<utag1,utag1_,SUBNET>;
|
|
template <typename SUBNET> using concat_utag2 = resize_and_concat<utag2,utag2_,SUBNET>;
|
|
template <typename SUBNET> using concat_utag3 = resize_and_concat<utag3,utag3_,SUBNET>;
|
|
template <typename SUBNET> using concat_utag4 = resize_and_concat<utag4,utag4_,SUBNET>;
|
|
|
|
// ----------------------------------------------------------------------------------------
|
|
|
|
static const char* semantic_segmentation_net_filename = "semantic_segmentation_voc2012net_v2.dnn";
|
|
|
|
// ----------------------------------------------------------------------------------------
|
|
|
|
// training network type
|
|
using bnet_type = dlib::loss_multiclass_log_per_pixel<
|
|
dlib::cont<class_count,1,1,1,1,
|
|
dlib::relu<dlib::bn_con<dlib::cont<64,7,7,2,2,
|
|
concat_utag1<level1t<
|
|
concat_utag2<level2t<
|
|
concat_utag3<level3t<
|
|
concat_utag4<level4t<
|
|
level4<utag4<
|
|
level3<utag3<
|
|
level2<utag2<
|
|
level1<dlib::max_pool<3,3,2,2,utag1<
|
|
dlib::relu<dlib::bn_con<dlib::con<64,7,7,2,2,
|
|
dlib::input<dlib::matrix<dlib::rgb_pixel>>
|
|
>>>>>>>>>>>>>>>>>>>>>>>>>;
|
|
|
|
// testing network type (replaced batch normalization with fixed affine transforms)
|
|
using anet_type = dlib::loss_multiclass_log_per_pixel<
|
|
dlib::cont<class_count,1,1,1,1,
|
|
dlib::relu<dlib::affine<dlib::cont<64,7,7,2,2,
|
|
concat_utag1<alevel1t<
|
|
concat_utag2<alevel2t<
|
|
concat_utag3<alevel3t<
|
|
concat_utag4<alevel4t<
|
|
alevel4<utag4<
|
|
alevel3<utag3<
|
|
alevel2<utag2<
|
|
alevel1<dlib::max_pool<3,3,2,2,utag1<
|
|
dlib::relu<dlib::affine<dlib::con<64,7,7,2,2,
|
|
dlib::input<dlib::matrix<dlib::rgb_pixel>>
|
|
>>>>>>>>>>>>>>>>>>>>>>>>>;
|
|
|
|
// ----------------------------------------------------------------------------------------
|
|
|
|
#endif // DLIB_DNn_SEMANTIC_SEGMENTATION_EX_H_
|